This vignette explains how to run a GOS
model in
spEcula
package.
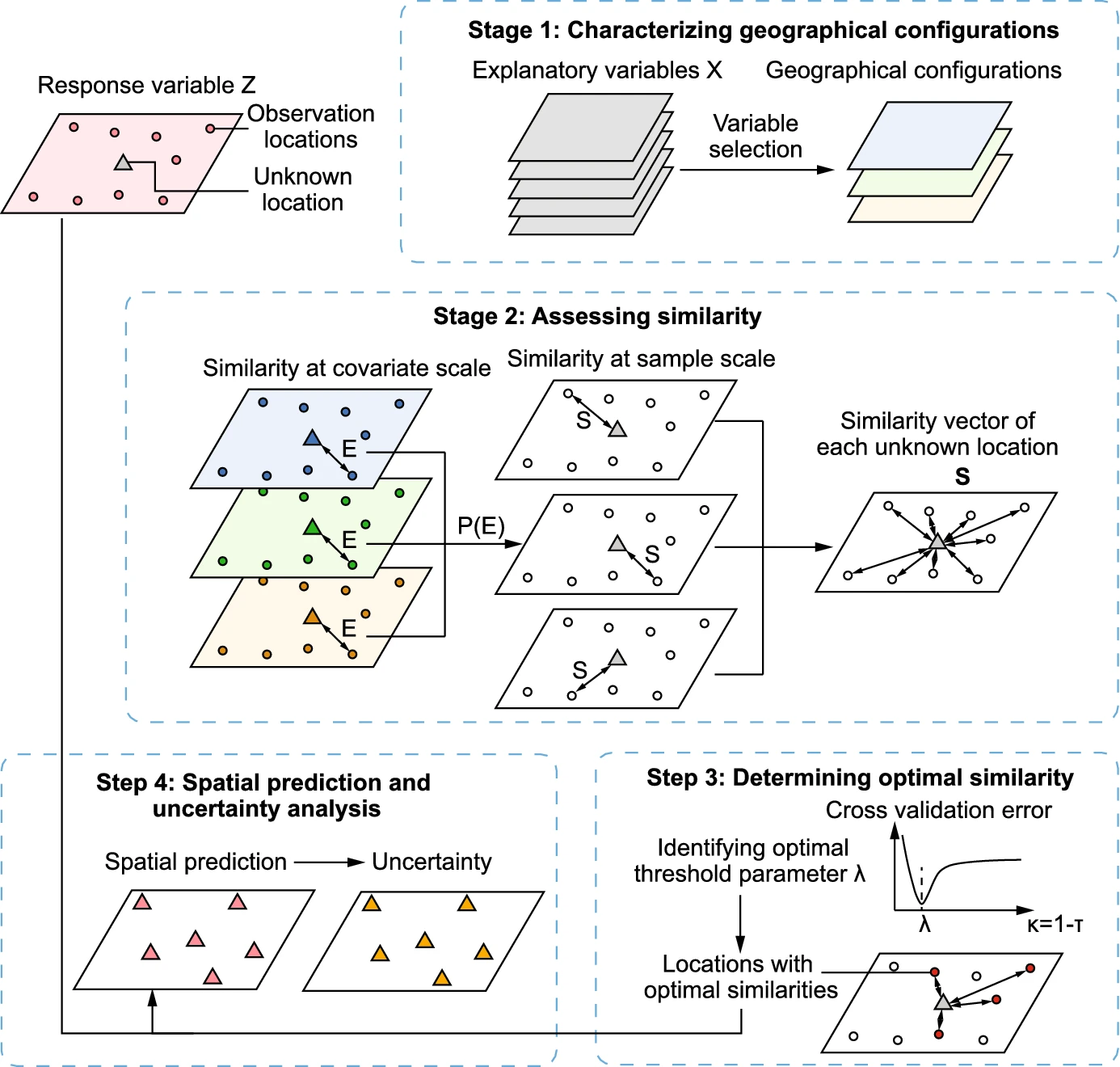
Schematic overview of geographically optimal similarity (GOS) model
Load data and package
use zn
data to train the gos
model,use
grid
data to predict.
Name | zn |
Number of rows | 885 |
Number of columns | 12 |
_______________________ | |
Column type frequency: | |
numeric | 12 |
________________________ | |
Group variables | None |
Variable type: numeric
skim_variable | n_missing | complete_rate | mean | sd | p0 | p25 | p50 | p75 | p100 | hist |
---|---|---|---|---|---|---|---|---|---|---|
Lon | 0 | 1 | 120.59 | 0.27 | 120.05 | 120.41 | 120.55 | 120.76 | 121.23 | ▃▇▇▃▃ |
Lat | 0 | 1 | -27.94 | 0.30 | -28.52 | -28.17 | -27.98 | -27.75 | -27.29 | ▅▆▇▃▃ |
Zn | 0 | 1 | 41.64 | 32.95 | 5.00 | 18.00 | 29.00 | 58.00 | 181.00 | ▇▂▂▁▁ |
Elevation | 0 | 1 | 481.54 | 34.70 | 398.89 | 462.64 | 485.56 | 507.04 | 562.99 | ▂▃▇▇▁ |
Slope | 0 | 1 | 0.30 | 0.15 | 0.01 | 0.20 | 0.27 | 0.39 | 0.81 | ▃▇▃▂▁ |
Aspect | 0 | 1 | 170.97 | 86.06 | 4.88 | 100.28 | 176.51 | 230.21 | 352.60 | ▅▅▇▅▃ |
Water | 0 | 1 | 0.67 | 1.06 | 0.00 | 0.05 | 0.15 | 0.80 | 6.41 | ▇▁▁▁▁ |
NDVI | 0 | 1 | 0.17 | 0.02 | 0.09 | 0.16 | 0.17 | 0.19 | 0.24 | ▁▂▇▆▁ |
SOC | 0 | 1 | 0.86 | 0.05 | 0.71 | 0.83 | 0.86 | 0.90 | 1.03 | ▁▅▇▃▁ |
pH | 0 | 1 | 5.73 | 0.17 | 5.28 | 5.63 | 5.75 | 5.85 | 6.12 | ▂▃▇▇▂ |
Road | 0 | 1 | 8.30 | 8.17 | 0.01 | 2.27 | 6.14 | 12.03 | 49.39 | ▇▃▁▁▁ |
Mine | 0 | 1 | 12.66 | 11.78 | 0.05 | 3.90 | 9.09 | 17.60 | 55.60 | ▇▃▁▁▁ |
skimr::skim(grid)
Name | grid |
Number of rows | 13132 |
Number of columns | 12 |
_______________________ | |
Column type frequency: | |
numeric | 12 |
________________________ | |
Group variables | None |
Variable type: numeric
skim_variable | n_missing | complete_rate | mean | sd | p0 | p25 | p50 | p75 | p100 | hist |
---|---|---|---|---|---|---|---|---|---|---|
GridID | 0 | 1 | 6566.50 | 3791.03 | 1.00 | 3283.75 | 6566.50 | 9849.25 | 13132.00 | ▇▇▇▇▇ |
Lon | 0 | 1 | 120.59 | 0.30 | 120.05 | 120.35 | 120.57 | 120.80 | 121.24 | ▆▇▇▅▃ |
Lat | 0 | 1 | -27.91 | 0.33 | -28.51 | -28.19 | -27.93 | -27.63 | -27.30 | ▆▇▇▆▆ |
Elevation | 0 | 1 | 482.70 | 36.70 | 398.05 | 463.23 | 485.13 | 508.37 | 588.65 | ▂▅▇▃▁ |
Slope | 0 | 1 | 0.28 | 0.16 | 0.01 | 0.17 | 0.25 | 0.35 | 1.71 | ▇▂▁▁▁ |
Aspect | 0 | 1 | 171.65 | 90.58 | 0.75 | 94.81 | 174.13 | 236.72 | 358.71 | ▅▆▇▆▃ |
Water | 0 | 1 | 1.11 | 1.19 | 0.00 | 0.26 | 0.65 | 1.60 | 7.70 | ▇▂▁▁▁ |
NDVI | 0 | 1 | 0.18 | 0.02 | 0.06 | 0.16 | 0.18 | 0.19 | 0.25 | ▁▁▆▇▁ |
SOC | 0 | 1 | 0.87 | 0.05 | 0.69 | 0.83 | 0.87 | 0.91 | 1.07 | ▁▅▇▃▁ |
pH | 0 | 1 | 5.74 | 0.18 | 5.11 | 5.63 | 5.75 | 5.87 | 6.18 | ▁▂▇▇▂ |
Road | 0 | 1 | 9.82 | 8.36 | 0.00 | 3.31 | 7.95 | 14.21 | 50.14 | ▇▅▁▁▁ |
Mine | 0 | 1 | 14.79 | 12.12 | 0.02 | 5.54 | 11.43 | 19.85 | 56.64 | ▇▅▂▁▁ |
Data pre-processing and variable selection
We will use the zn
data and grid
data o
predict Zn
in the scope of grid
data.
From above,we can see that zn
variable in
Zn
data is skewed (right skewed),so Let’s do a normality
test on it.
moments::skewness(zn$Zn)
## [1] 1.414892
shapiro.test(zn$Zn)
##
## Shapiro-Wilk normality test
##
## data: zn$Zn
## W = 0.84834, p-value < 2.2e-16
The Shapiro-Wilk normality test with a \(\text{p-value} < 2.2e-16 << 0.05\)
and W value of \(0.84834\), we can
conclude with high confidence that zn
variable in
Zn
data does not follow a normal distribution.
Now,we transform the zn
variable in Zn
data,here I use Power Transform
method.(ps: you can also
use a log-transformation). Power Transform
uses the maximum
likelihood-like approach of Box and Cox (1964) to select a
transformation of a univariate
or multivariate response for
normality. First we have to calculate appropriate transformation
parameters using powerTransform()
function of
car
package and then use this parameter to transform the
data using bcPower()
function.
lambdapt = car::powerTransform(zn$Zn)
lambdapt
## Estimated transformation parameter
## zn$Zn
## -0.02447525
zn$Zn = car::bcPower(zn$Zn,lambdapt$lambda)
Now, let’s see the transformed zn
variable in
Zn
data and see the skewness:
hist(zn$Zn)
moments::skewness(zn$Zn)
## [1] 0.004367706
All right, let’s move on to the next step to see variable correlation:
PerformanceAnalytics::chart.Correlation(zn[, c(3:12)],pch = 19)
and test multicollinearity use vif:
m1 = lm(Zn ~ Slope + Water + NDVI + SOC + pH + Road + Mine, data = zn)
car::vif(m1)
## Slope Water NDVI SOC pH Road Mine
## 1.651039 1.232454 1.459539 1.355824 1.568347 2.273387 2.608347
In this step, the selected variables include Slope, Water, NDVI, SOC, pH, Road, and Mine.
Determining the optimal similarity
tictoc::tic()
b1 = gos_bestkappa(Zn ~ Slope + Water + NDVI + SOC + pH + Road + Mine,
data = zn,kappa = c(seq(0.01, 0.1, 0.01), seq(0.2, 1, 0.1)),
nrepeat = 10,nsplit = .8,cores = 1)
tictoc::toc()
## 31.67 sec elapsed
b1$bestkappa
## [1] 0.07
b1$cvmean
## # A tibble: 19 × 2
## kappa rmse
## <dbl> <dbl>
## 1 0.01 0.610
## 2 0.02 0.602
## 3 0.03 0.597
## 4 0.04 0.596
## 5 0.05 0.594
## 6 0.06 0.594
## 7 0.07 0.594
## 8 0.08 0.594
## 9 0.09 0.594
## 10 0.1 0.594
## 11 0.2 0.594
## 12 0.3 0.594
## 13 0.4 0.594
## 14 0.5 0.594
## 15 0.6 0.594
## 16 0.7 0.594
## 17 0.8 0.594
## 18 0.9 0.594
## 19 1 0.594
b1$plot
You can set more optional numbers to the kappa
vector
and a higher value of the cross-validation repeat times
nrepeat
with a multi-core parallel(set cores
bigger).
tictoc::tic()
b2 = gos_bestkappa(Zn ~ Slope + Water + NDVI + SOC + pH + Road + Mine,
data = zn,kappa = c(seq(0.01, 0.1, 0.01), seq(0.2, 1, 0.1)),
nrepeat = 10,nsplit = .8,cores = 6)
tictoc::toc()
## 9.86 sec elapsed
b2$bestkappa
## [1] 0.07
b2$cvmean
## # A tibble: 19 × 2
## kappa rmse
## <dbl> <dbl>
## 1 0.01 0.610
## 2 0.02 0.602
## 3 0.03 0.597
## 4 0.04 0.596
## 5 0.05 0.594
## 6 0.06 0.594
## 7 0.07 0.594
## 8 0.08 0.594
## 9 0.09 0.594
## 10 0.1 0.594
## 11 0.2 0.594
## 12 0.3 0.594
## 13 0.4 0.594
## 14 0.5 0.594
## 15 0.6 0.594
## 16 0.7 0.594
## 17 0.8 0.594
## 18 0.9 0.594
## 19 1 0.594
b2$plot
Spatial prediction use GOS model
tictoc::tic()
g = gos(Zn ~ Slope + Water + NDVI + SOC + pH + Road + Mine,
data = zn, newdata = grid, kappa = 0.07,cores = 6)
tictoc::toc()
## 7.61 sec elapsed
back transformation using transformation parameters that have used Box-cos transformation
grid$pred = inverse_bcPower(g$pred,lambdapt$lambda)
grid$uc99 = g$`uncertainty99`
show the result
library(ggplot2)
library(viridis)
library(cowplot)
f1 = ggplot(grid, aes(x = Lon, y = Lat, fill = pred)) +
geom_tile() +
scale_fill_viridis(option="magma", direction = -1) +
coord_equal() +
labs(fill='Prediction') +
theme_bw()
f2 = ggplot(grid, aes(x = Lon, y = Lat, fill = uc99)) +
geom_tile() +
scale_fill_viridis(option="mako", direction = -1) +
coord_equal() +
labs(fill=bquote(Uncertainty~(zeta==0.99))) +
theme_bw()
plot_grid(f1,f2,nrow = 1,label_fontfamily = 'serif',
labels = paste0('(',letters[1:2],')'),
label_fontface = 'plain',label_size = 10,
hjust = -1.5,align = 'hv') -> p
p